Release time: 2020-05-04 11:40
Does Stanford Owe Us an Apology for that COVID-19 Study?
What was wrong with a recent study—and should they have known better?
A team of researchers from Stanford recently posted a preprint of a manuscript on COVID-19 cases that was wrong. Based on their results, they suggested that many more people had already had the virus than we realized. The upshot was supposed to be that maybe it didn’t cause symptoms as often as we thought, or that maybe we’d get to “herd immunity” more quickly and less painfully than we thought.
In a recent blog post, Columbia University statistician Andrew Gelman walks through problems with the analyses, and why they render the paper’s conclusions invalid. (Subsequent reporting brought to light other problems, such as the way participants were recruited and false promises made in recruitment.)
Beyond just saying that the paper is flawed, though, Gelman writes that the authors of the paper “owe us all an apology. We wasted time and effort discussing this paper whose main selling point was some numbers that were essentially the product of a statistical error.” Gelman is a critic of poor statistics generally, and he has pointed out fundamental flaws with several prominent psychology studies, too. As someone interested in science reform, I think it’s worth asking: Do these researchers *really* owe us an apology?
First, the criticisms. They’re technical statistical points, but I’ll outline three key ones so you can get the gist.
All tests for diseases are less than 100% accurate, and so you need to account for these in your statistics. For example, sometimes you get a “false positive” where the test says “you have this disease” but in reality you don’t. Based on the false positive rates identified in earlier research, you would expect up to 10% of the people in the study to test positive for COVID-19, even though they didn’t have it. In the Stanford study, this would mean you’d expect up to 333 diagnoses, even if no one had it. They only saw 50 diagnoses in their study. So basically all of the cases they saw could just be false positives, and you could have just as easily written up the study saying “there are potentially zero true COVID-19 cases in our whole study”—which is the opposite of their conclusion.
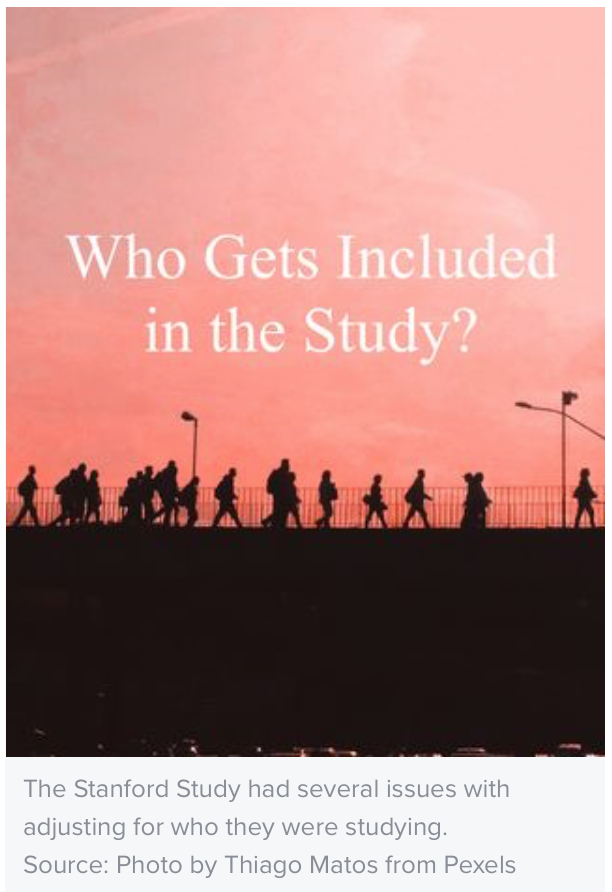
When you collect a sample, you don’t usually get the right proportions of people from each category. For example, only 5% of their sample was people over age 65, but in Santa Clara county, where they did the study, 13% of the people are over age 65. As Gelman writes, this is a “standard problem” and there are statistical adjustments that need to be made to account for them. The Stanford team didn’t adjust for age and they didn’t share the details of the adjustments they did make in a clear way. To make claims about how many people have it in the wider world, we need to take into account age. This is especially important with COVID-19, which might infect older people more.
Also related to who ended up being in the study: the study was advertised as a way to get free tests (and, relatedly, one ad falsely claimed that taking it could tell you whether you were safe to go back to work). That would bias who participates. My intuition is that it would pull for people who had symptoms or exposure, but the Stanford folks point out that it could just as likely have pulled for people who were healthy enough to travel to a testing site.
Gelman’s point is that they say they also collected info on other diseases and symptoms people were experiencing, and they could have done some statistical adjustments using that info—or even just described better the type of person who was seeking out the test. So it’s possible they were testing from people who were more likely to have COVID-19 than the general population, and this might have thrown their conclusions off.
It’s worth going through these issues, because they highlight just how much underlying technical knowledge of statistics is needed to do a study like this correctly. Doing science well and rigorously requires a lot of coordinated parts. The Stanford team reacted very quickly to the crisis, collecting a lot of data on this new virus and writing up the results in a relatively short time window. This is good—we want scientists rushing out to collect data on new problems society is facing. The coordination needed to complete the study was impressive, even if they didn’t have the statistical chops to come to the right ultimate conclusion.
But the Stanford team was also sloppy. They rushed to get their results out, but they released them into the world before they had been thoroughly vetted. The problem is related to the incentives in academic research. Getting a publication with flashy results out builds up the reputation of researchers and their institutions. The only way that a researcher can get and keep a job at Stanford is by repeatedly making bold, headline-grabbing findings. In informal discussion, a Stanford dean was reported to have said that “Stanford doesn’t give tenure for incremental research”—which means that you can’t have job security at Stanford if you’re doing careful, slow science that builds on what comes before. As psychologist Simine Vazire put it: “If you tell faculty they must focus on groundbreaking research, they might break a lot of other things besides new ground.”
One positive in this story is that the authors released their study as a preprint. Preprints are not peer reviewed journal articles, they’re drafts of papers that other scientists can read and comment on before they submit or publish the final, “official” version in a journal. That means that the public commentary on the manuscript will likely prevent its conclusions from being treated as True and being used to create policy. But if this was released as just a draft, shouldn’t Gelman be a bit easier on these folks, as they never claimed this was the last word? Isn’t it ok to get things wrong in a draft?
Ordinarily, I’d say that you should give people a pass on getting things wrong in their early drafts. But given that this research is being read by hundreds (maybe thousands) of people immediately to try to better understand what to do about COVID-19, it does seem troubling. For sensitive topics, I’d want researchers to be more careful. As Gelman notes, there are very good statisticians working at Stanford who they could have asked to read a draft. As Gelman also notes, this is one of a series of poorly done studies or misleading statements made by Stanford researchers during the COVID-19 pandemic, and each of these causes minor erosion to the institution’s reputation.
So should the researchers apologize? Maybe. But the larger lesson for members of the public who read scientific research should be to take into account the incentives at Very Prestigious Universities. Research coming out of Stanford and published at top scientific journals is more likely to be speculative and razzle-dazzle than research at middle tier institutions and in middle tier journals, just because Stanford is only likely to hire and promote people who prioritize that type of work. Maybe next time, we should look towards the less surprising, world-changing claims, and trust more modest claims made with humility.
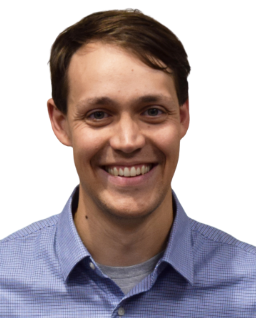
Alexander Danvers, Ph.D., a Postdoctoral Fellow at University of Arizona, researches emotions and social interactions.
Psychology today