Release time: 2020-10-28 15:05
The Exponential Growth of AI in Brain Care and Treatment
Italian researchers analyze more than a decade of applied AI for brain care.
Advances in computer science are helping to accelerate a broad spectrum of scientific research. The more complex the problem, the greater the potential for artificial intelligence (AI) machine learning to help identify patterns and make predictions. How widely is machine learning being used in treating diseases and disorders of the brain? A new study published earlier this month in the science journal APL Bioengineering examines the state-of-the-art uses of AI for brain disease, and shows there has been exponential growth in over a decade.
The biological brain has been the inspiration for artificial neural networks, a type of artificial intelligence (AI) machine learning model. Deep learning methods use artificial neural networks, and its pattern-recognition capabilities have contributed greatly to the current AI renaissance. Studying the brain often requires processing reams of complex imaging data, a daunting and time-intensive task for human scientists. Thus, machine learning can be a useful tool in the treatment of brain disorders and diseases.
In a study funded by Horizon 2020, the European Union’s EU Research and Innovation program, a team of Italian researchers from Politecnico di Milano and the University of Calabria set out to find out the different ways artificial intelligence is being used for brain care and identify the important clinical applications.
To achieve this, the team of Alice Segato, Aldo Marzullo, Francesco Calimeri and Elena De Momi queried 2696 scientific papers in the Pubmed, Scopus, and Web of Science databases dating as far back as January 1, 2008 using the search keywords of artificial intelligence and brain, which yielded 154 papers. The team then performed a systematic review of these scientific papers.
According to the researchers, there was “an exponential growth, in the latest ten years, of the number of studies evaluating AI models as an assisting tool across multiple paradigms of brain care,” and these paradigms include “diagnosis with anatomical information, diagnosis with morphological information, diagnosis with connectivity information, candidate selection for surgical treatment, target definition for surgical treatment, trajectory definition for surgical treatment, modeling of tissue deformation for intra-operative assistance, and prediction of patient outcome for postoperative assessment.”
The team found that AI was being used for patients for a variety of brain disorders such as Parkinson’s disease, brain tumors, epilepsy, cerebrovascular abnormalities, brain lesions, and brain injuries.
The types of algorithms were quite varied. These include regression algorithms, linear regression, logistic regression, instance-based algorithms, K-nearest neighbor (KNN), support vector machines (SVM), Bayesian algorithms, naïve Bayes (NB), clustering algorithms, K-means, Fuzzy C-means, hidden Markov model (HMM), sparse autoencoder (SAE), artificial neural network algorithms, deep learning algorithms, fully connected neural network (FCNN), convolutional neural network (CNN), corrective learning network (CLNet), recurrent neural networks (RNN), recurrent fuzzy neural networks (RFNN), long short-term memory networks (LSTM), deep belief networks (DBN), extreme learning machines (ELM), dimensionality reduction algorithms, linear discriminant analysis (LDA), ensemble algorithms, AdaBoost, random forest (RF), gradient boosting machines (GBM), gradient boosted regression trees (GBRT), sparse multi-view task-centralize (Sparse MVTC), genetic algorithms (GA), natural language processing (NLP), graph-based semi-supervision (GBS), multivariate analysis, and supervised LOCATE (locally adaptive threshold estimation).
A vast majority, 121 papers to be precise, used artificial intelligence for diagnosing brain disease and disorders. “This includes classification using anatomical information, morphological information, and connectivity information for neurological disorders, brain tumors, brain lesion, brain injury, Parkinson's disease, epilepsy and cerebral artery, schizophrenia, Alzheimer's disease, autism disorder, and multiple sclerosis," reported the researchers.
The most common pathology of the diagnosis was for brain tumors, neurological disorders, Alzheimer’s disease, and Autism disorder. The most commonly used data in the papers for diagnosis were mostly MRI (51 percent), and FC (31 percent). The AI methods most used are convoluted neural networks (30 percent), support vector machines (23 percent), random forest (12 percent), and artificial neural networks (7 percent).
“The use of artificial intelligence techniques is gradually bringing efficient theoretical solutions to a large number of real-world clinical problems related to the brain,” the researchers concluded. “Specifically, in recent years, thanks to the accumulation of relevant data and the development of increasingly effective algorithms, it has been possible to significantly increase the understanding of complex brain mechanisms.”
Copyright © 2020 Cami Rosso All rights reserved.
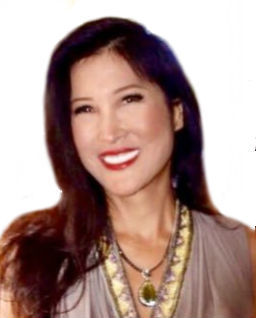
Cami Rosso writes about science, technology, innovation, and leadership.
psychology today